|
|
|
GillS posted on December 21, 2023 14:54
Well, the year seems to have flown by once again for us here at the EPPI Centre. Possibly because we have been super busy immersing ourselves in all things systematic review-related. Here is a round-up of the highlights of our year!
Shiny EPPI people!
This year we have welcomed two new team members and the return of a former EPPI colleague!
- Silvy Matthew joined the team for our new Evidence Synthesis Group (see below for details) – working across projects on screening for diabetic eye and on designing evidence synthesis methodology to capture contextual factors in a more helpful way for informing decision-makers.
- Hossein Dehdarirad is an information scientist whose primary interests are information retrieval and management, developing search strategies for evidence synthesis projects, recommender systems, text analysis, machine learning, as well as scientometrics.
- We’re also delighted to welcome back Mel Bond as a Research Fellow after a stint as a Lecturer (Digital Technology Education) at the University of South Australia.
We celebrated two of our team members whose contributions to the EPPI Centre and beyond, were recognised. Gillian Stokes became an Associate Professor of Inclusive Social Research and Rachael Edwards is now a Senior Research Fellow.
We were super-proud of our colleagues James Thomas, Rebecca Rees, Sandy Oliver, Mel Bond and Irene Kwan who were recognised for being in the top 2% of scientists in the world in the Elsevier and Stanford University’s 2023 list of the worlds most cited scholars of their field!
New reviews published in 2023
Work that has been published this year for the London-York NIHR Policy Research Programme Reviews Facility with our partners at the Centre for Research and Dissemination and the London School of Hygiene and Tropical Medicine, includes:
Please visit the EPPI Centre website for a comprehensive list of published reviews.
New ventures
London Alliance for the Coproduction of Evidence Synthesis (LACES): This year we were excited to launch LACES, which is one of nine Evidence Synthesis Groups funded across the UK by the NIHR. LACES is a collaboration of three UCL centres including the EPPI Centre, the UCL Co-Production collective and the Health Economics Policy Lab. The team will undertake a rolling programme of policy-commissioned reviews during a five-year period. Watch out for publications from LACES in 2024!
Much of our current work has proven to be very topical, such as our growing body of reviews around equity, machine learning and artificial intelligence. We are excited about publications on these topics in the coming year.
Key presentations
We were delighted to welcome the return of the Cochrane Colloquium in 2023, especially as it was on our home turf in London!
Here’s a few of the team’s workshops from this year’s colloquium…
And a few of our presentations…
Looking forward to 2024...
We’re already looking forward to catching up with national and international colleagues in September at the Global Evidence Summit in Prague!
And, we’re also very much looking forward to hosting some exciting presentations at the London Evidence Syntheses and Research Use Seminars which we run with colleagues at the London School of Hygiene and Tropical Medicine (LSHTM). Kicking off the 2024 series on 17th January, will be our very own James Thomas who will be discussing New 'AI' technologies for evidence synthesis: how do they work, and can we trust them? – we hope you can join us for this talk, either in person or online!
We hope that the festive season will be all that you hoped for and that 2024 will bring lots of exciting projects for you too.
Wishing you all a wonderful festive break!
|
Graziosi posted on December 19, 2022 15:02
For research teams everywhere, it seems the last few years since the COVID-19 pandemic began have been exceptionally busy. 2022 has been another very busy year for the EPPI Centre team. Gillian Stokes and Katy Sutcliffe look back over the year and share some of the highlights!
For research teams everywhere, it seems the last few years since the COVID-19 pandemic began have been exceptionally busy. 2022 has been another very busy year for us here at the EPPI Centre. So, we thought we’d look back over the year and share some of our highlights!
Shiny EPPI people!
This year it’s been wonderful to welcome three new team members.
We also celebrated one of our longest serving team members, Rebecca Rees, becoming a Professor of Social Policy and Head of the Social Science Research Unit (SSRU) where the EPPI Centre is based!
New reviews published in 2022
Reviews published this year for the London-York NIHR Policy Research Programme Reviews Facility with our partners at the Centre for Research and Dissemination and the London School of Hygiene and Tropical Medicine include:
We’ve also produced a number of reports for the International Public Policy Observatory on COVID-19. Including our most recent review on misinformation in COVID-19.
Please do visit our website for a comprehensive list of published reviews.
New methods work
Handling Complexity in Evidence from systematic reviews and meta-analyses of Public Health Interventions (CEPHI): This year we’ve been developing and testing four new methods for synthesizing evidence to better account for context than standard statistical meta-analysis approaches as part of this NIHR funded project. This work was underpinned by coproduction, and supported by the UCL Co-Production collective. Watch out for publications on this in 2023!
Examining the dimensions of equity (Research England Policy Support Fund): We are examining the methods used by systematic reviewers to conceptualise possible dimensions of health equity impacts of public health interventions, such as using a PROGRESS-Plus approach. These dimensions include socioeconomic status, race/ethnicity and gender/sex. We are also looking at the practical and conceptual issues encountered in applying such methods.
Key publications and presentations
We have been busy sharing our knowledge too.
Several team members contributed to and presented at a virtual conference in partnership with Manipal in India: International Conference on indigenizing systematic review evidence to local context for informing policy and practice decisions organized by Manipal College of Nursing, Manipal, Public Health Evidence South Asia, PSPH, MAHE in collaboration with Social Science Research Unit, Social Research Institute, University College London, UK.
We thoroughly enjoyed hosting a hub for the 2022 What Works Global Summit. The EPPI Centre team presented several papers and were delighted that the best session award went to a session on innovative approaches in evidence synthesis, which included three EPPI Centre presentations on the CEPHI project!
Here’s a few of the team’s publications from this year …
Looking forward to 2023 ...
New projects
We have two exciting new projects lined up for 2023!
A new round of funding from the International Public Policy Observatory means we’ll continue to contribute to reviews about the best ways to mitigate social harms associated with COVID-19.
We were also delighted to be successful in our bid to become one of the newly funded National Institute for Health and Care Research (NIHR) Evidence Synthesis Groups. We're extremely excited to be drawing on a range of cutting-edge methods to produce reviews during this five-year contract including: Engagement with stakeholders to ensure relevance; Co-production to ensure equity and inclusivity; Evidence-to-decision methods to ensure utility and impact; and Automation and continuous surveillance to ensure up-to-date evidence.
Events
We’re very excited that the 2023 Cochrane Colloquium will be on our home turf in London and hope to catch up with many people there!
And, we’re also very much looking forward to hosting some further excellent seminars as part of the London Evidence Syntheses and Research Use Seminars newly relaunched in collaboration with colleagues at the London School of Hygiene and Tropical Medicine (LSHTM). We’re trialling a hybrid format that we hope means we can connect with reviewers well beyond our London setting. Kicking off the 2023 seminars, will be a seminar on PPI in systematic reviews: challenges and possible solutions? On 25th January – we hope you can join us!
Wishing you all a wonderful festive break!
Image: "christmas tree lights" by pshab is licensed under CC BY-NC 2.0..
|
Graziosi posted on September 10, 2021 13:00
The concept of embedding researchers into policy and other settings is gaining traction as a way to enhance the role of research evidence in informing decision-making. A new EPPI Centre project explores the influence that 'embedded researcher' interventions could have in public health decision-making (see project page here). Embedded researcher interventions may be potentially useful in helping public health organisations to become more research active as they are challenged by widening health inequalities from COVID-19 and budget constraints. Early on in the project we have been confronted with a thorny issue – exactly what is an ‘embedded researcher’? In this blog Dylan Kneale, Sarah Lester, Claire Stansfield and James Thomas discuss this challenge and why researching this ‘intervention’ is important, and we are interested in your feedback on this.
By Dylan Kneale, Sarah Lester, Claire Stansfield and James Thomas.
Introduction
Embedding researchers into policy and other settings is thought to be a way of enhancing research capacity within organisations to enable them to become more involved in the research process either as consumers, generators, commissioners, influencers, stakeholders or a mixture of these roles. The literature suggests that research evidence could have a more prominent role in public health decision-making than is currently the case1 2. In our project, we view embedded researchers as potential disruptors of organisational cultures, who can enable organisations to become more active through occupying roles as mobilisers or (co)producers of research, or facilitators of research use. Embedded researchers could address these issues in a number of ways including through (i) actively researching and contributing to decision-making processes; (ii) changing cultures around research engagement, including the indirect or diffuse influence of research (enlightenment), direct research utilisation (instrumental usage), or research generation; and (iii) helping to produce research evidence that matches the need of decision-makers with regards to the research questions asked and their contextual salience. Part of our project involves systematically identifying research evaluations of embedded research across a range of policy, industry and commercial settings in order to produce a systematic map and a systematic review. Developing a set of principles to define an embedded researcher is crucial to this goal, as existing definitions of embedded research do not fully capture what we are seeking to achieve.
Existing definitions of embedded researcher
Embedded researchers within local public health decision-making contexts have previously been defined as those ‘researchers who work inside host organisations as members of staff, while also maintaining an affiliation with an academic institution’3. McGinty and Salokangas (2014)4 define embedded researchers as: “individuals or teams who are either university-based or employed undertaking explicit research roles […] legitimated by staff status or membership with the purpose of identifying and implementing a collaborative research agenda.” Meanwhile they define embedded research as: “a mutually beneficial relationship between academics and their host organizations whether they are public, private or third sector” (McGinity & Salokangas, 2014, p.3). While these definitions are unproblematic in many ways, the extent to which this definition captures the plurality of models of embedded research is unclear, and the extent to which some of the models of placement where researchers may not feel they have, or may not be recognised as having, dual affiliation is unknown. This is of significance for our systematic map and review. For example if applied literally, the first definition may serve to omit forms of embedded research activity undertaken by researchers who may be based in a host (non-academic) organisation but may not be considered ‘staff’ (e.g. PhD students); meanwhile the second definition, if applied literally, could lead to very short term activities such as delivering research training, being in scope. These ambiguities appear to suggest that ‘embedded researcher’ appears as a concept that we may know when we see it, although is challenging to define precisely.
Why is defining an embedded researcher so challenging?
Some of the reasons why defining embedded researchers is so challenging include:
- Terminology: Embedded researchers, researchers-in-residence, seconded researchers, policy-fellows, embedded knowledge mobilisers, and several other terms appear to be aligned with the focus of our project.
- Intervention complexity: While embedded research activities are rarely broken down into intervention ‘components’ per se (an aim of our project), we would nevertheless expect that an embedded researcher intervention would have several interacting ‘components’, with change occurring in non-linear ways that are manifested across different organisational roles and levels, and that there would be a high degree of tailoring across embedded researcher ‘interventions’ 5; these properties are synonymous with intervention complexity.
- Fuzziness: Not only is an ‘embedded researcher’ a fuzzy concept in itself; it is also predicated and defined through a number of other fuzzy concepts (e.g. ‘research-active’, ‘organisational change’, ‘research skills’ etc.) each of which is open to interpretation.
- Patterns of evaluation: Although embedded research activities have a long tradition, our initial assessment of the literature suggests that these interventions tend to be evaluated through self-reflection and descriptive case studies. Although these are useful, richer evaluation data being made available would help to understand impacts of embedded researchers.
- Non-manualised: Related to the points above, embedded research ‘interventions’ tend to take place without any form of ‘manual’ or ‘handbook’ on how the intervention should be conducted.
Towards a set of principles defining embedded research activity
Rather than a crisp neat definition, embedded research activity may instead be more usefully defined through a set of principles. With the input of our steering group, we view embedded researchers as being defined by the following principles:
(i) they enable research activity and research use. For example, they may undertake research, facilitate the conduct of research (through sourcing data, creating data sharing arrangements or advising on research processes), and support research use;
(ii) they are co-located in a defined policy, practice or commercial formal organisation;
(iii) they are situated within a host team (physically or institutionally or affiliation or culturally) and/or are expected to work within the host team culture for a high proportion of their time as a team member working on and solving practical problems;
(iv) they have an affiliation with an academic institution or research organization, or their post is specifically funded by an academic institution or research organization;
(v) these activities entail continued engagement with a host team (i.e., an embedded researcher is more than a notional job title but a different way of working for researchers);
(vi) the relational nature of embedded research necessitates that this is a long-term activity (i.e., unlikely to be possible in less than a month);
(vii) we expect that host organisations will be able to influence and direct the work of embedded researchers (i.e., embedded researcher activities are generally distinct from, for example, an ethnographic study of policy-making in an organization);
(viii) we view embedded researchers as contributing pre-existing skills and experience to the host organization, and not developing these skills on-the-job and therefore we do not view taught degree placements as examples of embedded researcher activities;
finally, and crucially (ix) we view embedded researchers as exemplifying a two-way relationship where there is learning to be gained for both embedded researchers and their host organisations. Our definition also leaves open the possibility of bi-directionality in that researchers could be embedded into policy/practice settings and that those from policy/practice settings could be embedded into research organisations (provided they meet the other principles).
Why is studying embedded researcher activity important?
Embedded researchers may be in a pivotal position to actively span boundaries between policy organisations and research organisations. This may be important as we emerge from a period where Local Authority public health teams are being asked to do more with less, having been besieged by cuts to budgets pre-dating the COVID-19 pandemic6, and where public health systems have been under massive strain during the current pandemic. Our research is also taking place in the context of new proposed changes in health systems and delivery structures with a focus on further integration of public health, ‘clinical’ health (NHS), and social care (among other proposed changes7), and further changes in the organisational structure of public health and the abolishment of Public Health England8, requiring greater consideration of the role of embedded researchers within integrated teams.
As a team we are also interested in whether embedded researcher interventions can help to address an emerging gulf in terms of health inequalities. While our understanding of the extent and nature of health inequalities appears to be increasing, this understanding has generally not (i) mitigated the increasing gap in health outcomes between different social groups; or (ii) kept pace with our understanding of if/how health inequalities can be addressed at the local level. We are interested if embedded researchers help to address these gaps and how.
Why have we written this blog?
If you’ve read this blog to this point – thank you! – and our intention in writing this blog is to reach out to ask for your input in the early days of this project. Please get in touch with us (email D.Kneale@ucl.ac.uk) if you would like to help in any of the following ways:
- Comment! Let us know what you think of our definition of embedded researchers
- Alert us! Let us know if you have any studies that you think should be included in a review of embedded researcher activity, or if you are working on relevant research in this area.
- Get involved! We are currently scoping out an evaluation study of embedded research activity. If you are working as an embedded researcher in public health, or are planning to do so, please let us know. We would love to talk to you about this.
References
1. Kneale D, Rojas-García A, Raine R, et al (2017). The use of evidence in English local public health decision-making. Implementation Science, 12(1):53.
2. Orton L, Lloyd-Williams F, Taylor-Robinson D, et al (2011). The use of research evidence in public health decision making processes: systematic review. PloS One, 6(7):e21704.
3. Vindrola-Padros C, Pape T, Utley M, et al. (2017) The role of embedded research in quality improvement: a narrative review. BMJ Quality and Safety, 26(1):70-80.
4. McGinity R, Salokangas M (2014) Introduction:‘embedded research’as an approach into academia for emerging researchers. Management in Education 28(1):3-5.
5. Thomas J, Petticrew M, Noyes J, et al. (2019) Chapter 17: Intervention complexity. In: Cochrane Handbook for Systematic Reviews of Interventions (2nd ed) Chichester (UK): John Wiley & Sons.
6. Iacobucci G (2016) Public health—the frontline cuts begin. BMJ, 352.
7. Iacobucci G (2021) Government to reverse Lansley reforms in major NHS shake up: British Medical Journal Publishing Group.
8. Vize R (2020) Controversial from creation to disbanding, via e-cigarettes and alcohol: an obituary of Public Health England. BMJ 371.
|
Graziosi posted on April 12, 2021 12:22
Over the past year we have seen what may euphemistically be described as “ambitious” uses made of predictive modelling to inform public policy. This is not a new phenomenon, but an established direction of travel. Unfortunately, such models have epistemic limitations that no amount of optimism can overcome. Assigning individuals to specific categories – with direct consequences for their lives and without recognising uncertainty in the prediction – is unsupportable scientifically and ethically. It ignores intrinsic uncertainties, reinforces existing structural disadvantage, and is inherently and irredeemably unfair. Model-based predictions may have an important role to play but, despite advancements in technology and data, we still need to exercise great caution when using predictions to place individuals in categories with real-world consequences for people's lives.
By James Thomas and Dylan Kneale
‘Scientific’ prediction is viewed as a necessary precursor for, and often a determinant of, public policy-making (Sarewitz & Pielke Jr, 1999); without some form of prediction or forecasting of the likely impacts of policy decisions, decision-makers may find themselves paralysed by inaction or shooting in the dark. Rather than gazing into crystal balls, decision-makers can draw on an increasingly sophisticated array of data and methods that are used to generate predictions. There is a lot of enthusiasm for these predictions, and some have claimed that they have the skills to forecast future events precisely; that they are ‘super-forecasters’ (Kirkegaard, Taji, & Gerritsen, 2020). However, while new possibilities are indeed opening up, we need to be mindful of the assumptions that underpin statistical models, and of the fact that large datasets and sophisticated statistics cannot perform miracles, simply because they are large and sophisticated. ‘Super-predictors’ are still subject to basic evidential laws that cover all research.
This is a wide-ranging field, although the issues we focus on here mainly reflect the epistemic impossibilities of using model-based predictions indiscriminately to allocate individuals into discrete categories of real-world significance, without acknowledgement of the uncertainty in doing so.
The implication that arises is that, where uncertainty cannot be incorporated in a real-world use scenario, then the use of the model in that scenario is inadvisable and manifestly unethical. This blog is prompted by recent examples in UK educational policy where there have been attempts to extrapolate model-based predictions to allocate individuals into categories, as well as debates in public health suggesting that there is widespread potential for predictions to be used in this way. These are not isolated examples and there are examples across public policy where model-based predictions have been used to make decisions about individual outcomes; for example model-based predictions of recidivism in the US have been used by judges in decisions about whether to release or hold a defendant before trial (Dressel & Farid, 2021)*. The (mis)use of predictive models and algorithms to ‘objectively’ and deterministically categorise individuals into discrete categories of real-world significance leads us to regard such exercises as attempts to escape epistemic gravity with optimism.
Extrapolating predictions to individuals
Models used for prediction purposes are of huge value across several areas of public policy and practice. Some predictions may have reasonable predictive power for groups of people with particular social profiles (e.g. socioeconomically disadvantaged vs advantaged people), but don’t travel well when applied to individuals. This can be problematic for at least six reasons:
The first, most fundamental issue is that predictions are made on the basis of probabilistic/stochastic models, but the classification of individuals into real-world categories is deterministic and crisp. A recent example of the flaws in this reasoning involved the use of predicted grades in the UK during the COVID-19 pandemic in 2020 to estimate children’s academic achievement. When predicting an exam grade based on a regression model, there would be uncertainty around the prediction itself, and the model would include an error term that denoted the unexplained variance. This uncertainty is ignored when the prediction is extrapolated from the model to allocate an individual grade to a real-world person, and the ‘fuzziness’ of the prediction is ignored. This is despite the range of uncertainty when predicting a (new) individual value from a regression model being wider than the range of uncertainty when interpreting a statistical parameter from a model, because of the added uncertainty in predicting a single response compared to a mean response. In the case of A-level and GCSE exams, a more accurate way of presenting results may have been to put intervals reflecting uncertainty in prediction around them. However, this would have resulted in the slightly bizarre situation where someone, for example, might have been predicted and awarded a grade ‘between A and C’. This is clearly not operational in practice, especially as some students could be allocated grades spanning the entirety of grades A-E, but it would have exposed the inherent uncertainty in the model; something that was concealed by the crisp prediction.
Secondly the predictions, and certainly the approaches underlying the creation of the predictions, may not have been developed with the intention of being applied to wide swathes of the population indiscriminately, and to assign individuals to categories of real-world significance. Instead, the logic underlying these models may hold that predictions from models be useful as diagnostic aids, but not the sole determinant of individual classification. If a prediction is to serve as a diagnostic aid, and not the sole basis of individual classification, the prediction may need to be based on, or combined with, additional information about the individual.
The third issue is that even when we have reliable prior information about an individual, using this in a prediction does not reduce error to non-trivial levels. In the case of predicting grades based on previous attainment for example, we are only able to do this with limited accuracy (Anders, Dilnot, Macmillan, & Wyness, 2020). Nevertheless, in the case of last summer’s school grades debacle, models incorporating past achievement at school level were treated as being entirely deterministic, with zero error – not a defensible position. Furthermore, the accumulating evidence demonstrating how poor we are at predicting individual grades undermines the entire idea that predicting individual academic achievement has analytic value, and suggests that the widespread use of individual predictions in the education system is outdated (see twitter thread by Gill Wyness and paper by Anders et al. (2020)).
The fourth issue is that increasingly sophisticated modelling and technologies can engender false confidence in our ability to predict. A recent example involves the concept of Precision Public Health (PPH), the topic of a new report by the EPPI-Centre, which refers to use of novel data sources and/or computer science-driven methods of data analysis to predict risk or outcomes, in order to improve how interventions are targeted or tailored. Our work on PPH suggests that where evidence is generated using new methods of analysis (specifically Artificial Intelligence) and/or new forms of data, that the findings from this evidence have a tendency to be interpreted by others to support claims about improvements in accuracy in ways that overreach the findings in the original study. Alongside this, we also observed that many of the new ways of analysing data and creating predictions about who or where may benefit most from a given Public Health intervention had not been evaluated against performance from more established methods. Certainly in the case of some analytical techniques, there is conflicting evidence around whether new approaches, such as those used in machine learning, can and do outperform traditional analytical methods such as logistic regression in prediction models (Christodoulou et al., 2019).
A fifth issue revolves around measurement error in the variables and other inaccuracies in the composition of the data used to generate the prediction, including missing data. Although these issues represent caveats to most if not all quantitative social science, the implications for model-based predictions may be unexpected.
A sixth issue is the potential for predictions to reinforce existing systemic biases and disadvantages. Returning to the example of school grades, there is a good deal of evidence demonstrating that the final grades that young people achieve in schools reflect systematic disadvantages faced by poorer and minoritised groups (Banerjee, 2016). A ‘perfect’ prediction model would be one able to replicate the exact results of ‘average’ (non-COVID-19) school years, which would include replicating these systemic inequalities and biases. The subsequent use of these models to predict future outcomes for individuals means that inequalities persist and are actually reinforced by prediction models. For instance, with respect to the earlier example of recidivism, a widely used tool to generate model-based predictions of recidivism in the US systematically overestimated the risk of black defendants re-offending and underestimated the risk of re-offending for white defendants (Dressel & Farid, 2021).
Allocation of individuals into discrete, ‘neat’ categories, on the basis of an ‘objective’ model, belies both the fuzziness of models and the structural disadvantages and structural racism that are underlying features of the models. This was one of the concerns also expressed about the direction of travel of Precision Public Health, where predicted differences in health were at risk of being interpreted almost as ‘innate’ and static features of individuals and attributable to individual factors, thereby overlooking structural and systemic disadvantages that give rise to these differences. Using predictions from models that reflect an unequal society, to predict outcomes for individuals, risks perpetuating further inequalities and undermines claims of objectivity, if the underlying model unquestioningly reproduces and perpetuates the subjectivities and biases of society at large, when categorising individuals.
Concluding points
Predicting outcomes for individuals based on population-level models and treating the source models (and the prediction) as having zero error is an epistemic impossibility. Similarly, treating error from a model as constant and fixed over time, contexts, and populations is also clearly problematic and not supported by evidence. However, the issue is often less to do with the robustness of the underlying model, but more a reflection of the mismatch between the underlying model assumptions and the ambition for real-world individual-level applications of the prediction.
Regardless of their ostensible superiority, we should approach even the most sophistically derived predictions for individuals with a critical eye with regards to the issues outlined above. AI-based predictions can sometimes be viewed as authoritative because of the putative improvement in the precision of the prediction, based on the novelty of the data and complexity and perceived objectivity of the algorithms used to generate them. Our own work around PPH emphasised that there is merit in both (i) questioning the rationale for prediction, and (ii) questioning the evidence base supporting the prediction for individuals (Kneale et al., 2020).
Over the year we have seen the phrase ‘following the science’ bandied about by policy-makers, and often directed at the use of predictions of likely scenarios that could follow in terms of the spread of the COVID-19 pandemic. The narrative of politicians during the COVID-19 pandemic has been one where model-based predictions, albeit ones directed at a population-level, have been viewed as a form of instrumental evidence to base policy decisions upon. However, because of the degree of uncertainty involved in generating these predictions, they may instead be at their most helpful in further illuminating the landscape within which policy decisions are made, rather than for instrumental use.
In the case of predicted grades, the literature was clear for some time before that predictions of future grades for individuals are far from accurate. The aftermath provides an opportunity to think again about how we use individual predictions in the education system, but perhaps more broadly across policy domains. Fundamentally, we should accept that it is simply impossible to use these models deterministically to allocate individuals to crisp categories with real-world consequences: we cannot overcome epistemic gravity with heroic optimism.
About the authors
James Thomas is professor of social research & policy and deputy director of the EPPI-Centre. His interests include the use of research to inform decision-making and the development of methods, technology and tools to support this.
Dylan Kneale is a Principal Research Fellow at the EPPI-Centre. He is interested in developing methods to enhance the use of evidence in decision-making, focusing on public health, ageing and social exclusion.
Notes and references
*The types of model-based prediction of most concern here are those that involve prediction of a future state or event which may be observed under normal conditions or with the passing of time, and less so those that involve ‘prediction’ of a latent (unobserved) contemporaneous state (which reflect more a form of categorisation than prediction).
Anders, Jake; Dilnot, Catherine; Macmillan, Lindsey & Wyness, Gill. (2020). Grade Expectations: How well can we predict future grades based on past performance? Centre for Education Policy and Equalising Opportunities, UCL Institute of Education, University College London.
Banerjee, Pallavi Amitava. (2016). A systematic review of factors linked to poor academic performance of disadvantaged students in science and maths in schools. Cogent Education, 3(1), 1178441.
Christodoulou, Evangelia; Ma, Jie; Collins, Gary S; Steyerberg, Ewout W; Verbakel, Jan Y & Van Calster, Ben. (2019). A systematic review shows no performance benefit of machine learning over logistic regression for clinical prediction models. Journal of clinical epidemiology, 110, 12-22.
Dressel, Julia & Farid, Hany. (2021). The Dangers of Risk Prediction in the Criminal Justice System. MIT Case Studies in Social and Ethical Responsibilities of Computing.
Kirkegaard, Emil Ole William; Taji, Wael & Gerritsen, Arjen. (2020). Predicting a Pandemic: testing crowd wisdom and expert forecasting amidst the novel COVID-19 outbreak.
Kneale, Dylan; Lorenc, Theo; O'Mara-Eves, Alison; Hong, Quan Nha; Sutcliffe, Katy; Sowden, Amanda & Thomas, James. (2020). Precision public health – A critical review of the opportunities and obstacles. London: EPPI-Centre, Social Research Institute, University College London.
Sarewitz, Daniel, & Pielke Jr, Roger. (1999). Prediction in science and policy. Technology in Society, 21(2), 121-133.
Image source, by Emir ÖZDEMİR, pixabay license.
|
Graziosi posted on October 03, 2018 12:54
EPPI-Reviewer is the software tool developed and used by those at the EPPI-Centre to conduct Systematic Reviews. It is also offered as a service for the wider Evidence Synthesis research community. At the same time, it plays a critical role in enabling methodological innovation. James Thomas and the EPPI-Reviewer team share an insight on their current development priorities as well as the general philosophy that drives their effort.
This is the first in a series of blogs about EPPI-Reviewer. We have many exciting developments in the pipeline and will use this blog to let you know about them over the next few months.
This blog piece sets the scene. It gives a little flavour of the development philosophy behind the software, and some of our current development priorities.
Development philosophy
EPPI-Reviewer grew out of a need within the EPPI-Centre for bespoke software to support its reviewing activity. We have always been interested in methodological innovation and needed a tool that would support this. We also conduct systematic reviews in a wide range of subject areas and using a range of different types of research. We therefore needed a flexible tool that could support the breadth of reviews that we undertook. We were not the only people who needed such a tool, and as requests came in for other people to use the software, we developed what is now the EPPI-Reviewer service. The current version of EPPI-Reviewer (version 4) first went online in 2010, and we have extended its functionality substantially since. (For those of you interested in history, EPPI-Reviewer began as a desktop application in 1993 called ‘EPIC’ and first went online as a service to people outside the EPPI-Centre in the late 1990s.)
So, EPPI-Reviewer is designed from the bottom up to be a flexible research tool that supports a range of methodologies – and also methodological innovation. The development team is in close contact with colleagues conducting reviews (in the same small building as many) so we have been able to extend and modify the software in response to reviewer need. For example, it contains the widest range of automation functionality of any systematic review tool (supporting ‘priority screening’ for efficient citation screening, with three high performing study-type classifiers, the ability to ‘make your own’ machine learning classifier, and text clustering tools); it supports meta-analysis, meta-regression and network meta-analysis using packages in the ‘R’ statistical environment, as well as ‘line-by-line coding’ for qualitative evidence synthesis. Packing such a large range of functions into a single application is challenging of course, and we have prioritised functionality over providing a smooth ‘workflow’ model, where reviewers are expected to follow a specific path.
Current technology challenges
EPPI-Reviewer version 4 runs in the Microsoft Silverlight browser plugin. This provided a ‘desktop-like’ application experience in a web browser across the Windows and OSX operating systems. The demise of such plugins and the rise of diverse mobile devices has led to a rapid development effort. We launched the first version of a new user interface last month: a tool which mimics the ‘coding only’ functionality that you may be familiar with. This enables users to do screening and data extraction in any web browser without the Silverlight plugin.
Currently, it is necessary to do the review setup and analysis phases using the Silverlight version (for practical guidance re Silverlight, please see here), so we are now extending our new user interface to support these processes too – and hope to have most of the essentials covered by Christmas. The figure below shows how, in the meantime, we will have two different user interfaces interacting with the same database. This means that you can log in using either user interface and work on exactly the same review.
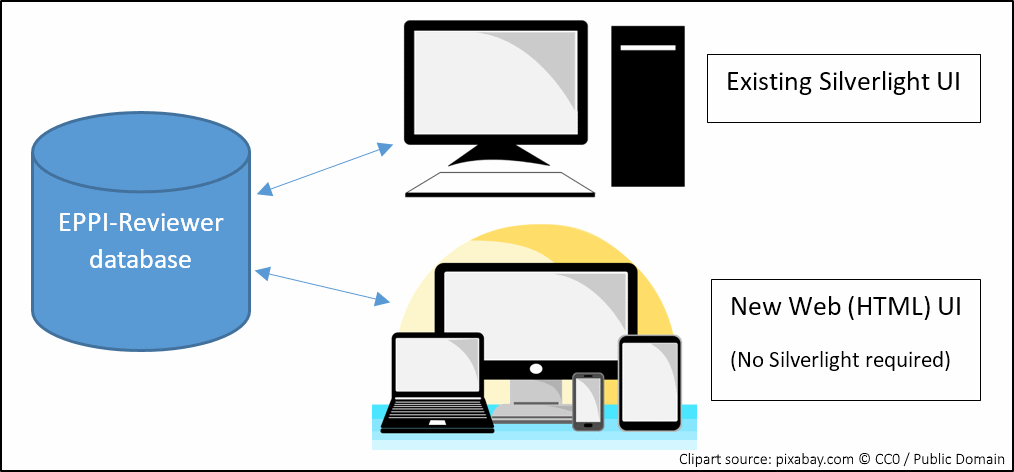
New and upcoming developments
We have partnered with the Campbell Collaboration to produce a user interface – based on the EPPI-Reviewer database – for ‘mapping’ research activity. These maps provide a user-friendly way of presenting the extent and nature of research in a broad domain. The columns and rows in the example below are defined in EPPI-Reviewer as ‘codes’ and the circles indicate the quantity and quality of evidence in each cell.
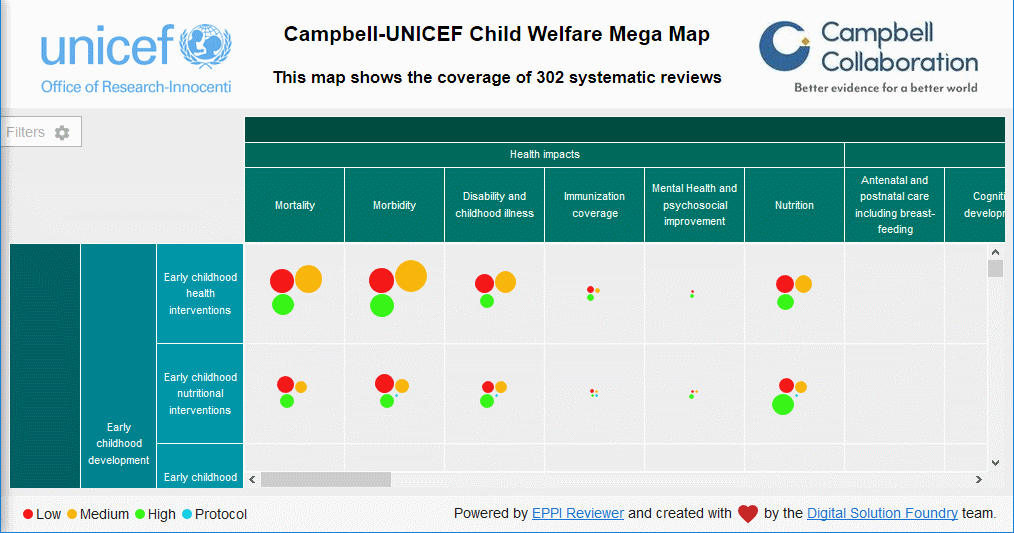
We are also designing some new data services as application programming interfaces (APIs) that a range of different applications can use. These data services are built on large sets of data and incorporate the latest automation tools to enable users to locate relevant research quickly. These services are looking forward to a world of ‘living’ systematic reviews (which we have written about elsewhere) where we keep reviews updated with new evidence as soon as it becomes available. We are planning to extend our support for ‘maps’ to include creating automatically updated maps in due course. Dealing with large amounts of data poses significant technical challenges, but being researchers, we are also busy evaluating the strengths and weaknesses of underpinning reviews with new data services. When we make these services available, we will also need to publish robust evaluations to support their use.
What about EPPI-Reviewer Version 5?
As some of you may know, we have been partnering with NICE to develop a new version of EPPI-Reviewer. The software is now being rolled out internally at NICE, but is currently more attuned to NICE’s needs than other external users, so we are concentrating our immediate development effort on giving our users an alternative entry point into the existing EPPI-Reviewer database. This strategy also gives users a seamless migration from one user interface into the other.
Conclusion
As this brief post indicates, this is a busy and exciting period of development here at the EPPI-Centre. We are always busy making progress on multiple fronts, so to make sure we can rapidly adapt our plans as and when we discover what works best for us and our whole community. As always, this means that feedback received via the forums and email is highly appreciated. As we move forward we will post further updates. Please do get in touch if you have any questions or feedback.
The EPPI-Reviewer Core team
The EPPI-Reviewer core team is small, agile and tight. The main members are listed below, in order of appearance. This list does not include the numerous people in the EPPI-Centre and beyond, who on occasion provide suggestions, advice and/or specialised contributions – this other list would be much, much longer!
James Thomas is the team leader and scientific director – he wrote the very first version(s) of EPPI-Reviewer and produces the prototypes of most new/innovative features of EPPI-Reviewer. James also leads the methodological-evaluation efforts that normally precede making any new methodology available to the larger community.
Jeff Brunton oversees user support and licensing. He is the lead developer for the Account Manager, the Web-Databases applications, RIS export and directed the development of the new mapping tool – along with James, he wrote EPPI-Reviewer version 3. He is also responsible for Testing and User Experience.
Sergio Graziosi spends most of his time inside the EPPI-Reviewer code. He brings James’ prototypes to production and makes sure all nuts and bolts are tightened up, while mechanisms (including systems) are well oiled. Along with Patrick, he wrote the first version of the (non-Silverlight) coding App.
Zak Ghouze provides user support and looks after our numerous systems. He is also consulting in designing the shape and feel of the new interface as well as providing invaluable insight into the minds of EPPI-Reviewer users. Along with Jeff, he is shaping the User Experience of our latest developments.
Patrick O’Driscoll writes code for all occasions. He brings fresh ideas to the team by means of new and varied experience on Web-Based front-ends as well as vast array of technologies and development methodologies. Together with Sergio, he is now busy writing the new web-based application.
|
Graziosi posted on October 02, 2018 14:03
Enthusiasm for public and patient involvement (PPI) in research is gaining momentum. But how can stakeholders be involved in a review process? What are the specific arguments and challenges for involving young people who are deemed to be ‘vulnerable’ in the research that affects them? Louca-Mai Brady and Sarah Lester reflect on the key lessons learned from holding a workshop for young people with lived experience of adverse childhood experiences in the early stages of a review.
What is the review about?
Adverse Childhood Experiences (ACEs) are stressful experiences that happen during childhood or adolescence that directly harm a child or negatively affect the environment in which they live. It is estimated that almost half of adults in England have been exposed to at least one form of adversity during their childhood or adolescence. A large US study in the 1990s first popularised the term ACEs and explored the negative impact of unaddressed childhood adversity on people’s health and behaviour across the life course. More recent research suggests that at least one in three diagnosed mental health conditions in adulthood directly relate to trauma brought about by ACEs.
The alarming prevalence and consequences of ACEs are largely understood, but the Department of Health and Social Care want to know what helps improve the lives of people with experience of ACEs. We are currently conducting a review of reviews to try to answer this question as part of the Department of Health and Social Care Reviews Facility. Our definition of ACE populations is informed by the previously mentioned US study and the UCL Institute of Health Equity’s ACE review. It spans twelve distinct but interrelated populations.
|
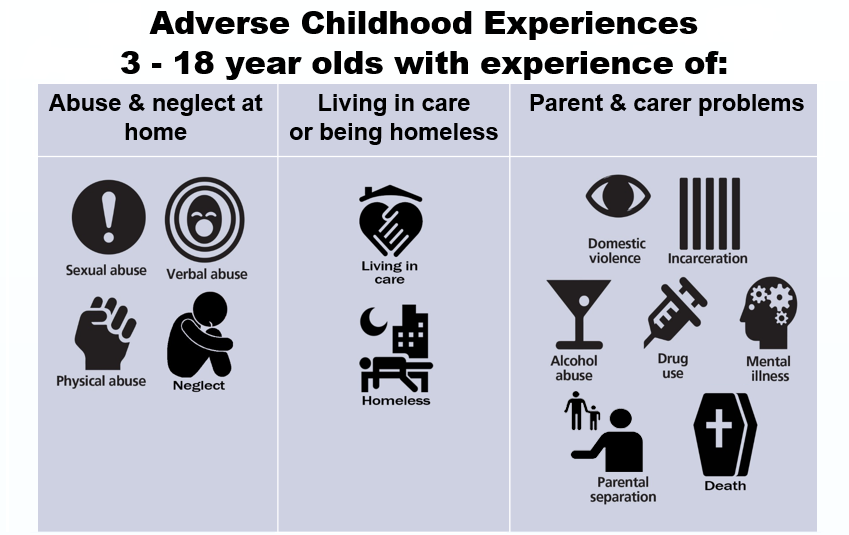 |
Populations as defined for the ACEs review |
Why did we want to involve young people in the review?
The 1989 UN Convention on the Rights of the Child established international recognition that all children have a right to have a say in decisions that affect them.
However, the voices of young people who are deemed to be ‘vulnerable’ are often absent from the literature and consequently there is the risk that they will be underrepresented in the key outputs designed to inform policy (see findings from the UN Committee on the Rights of Child, UNRC, 2016, p.6-7). Involvement should also lead to research, and ultimately services, that better reflect young people’s priorities and concerns (Brady et al., 2018). With this in mind, we wanted to consult with young people with lived experience of ACEs in order to keep our review grounded in their experience and perspectives.
We decided to hold a workshop during the early stages of the review process to help us to verify whether the evidence we were finding was relevant to the current UK context and young people’s lived experience, and to explore how we might involve young people later on in the review if possible, or in future research.
While involving children and young people in research is still an emerging field (Parsons et al. 2018), the work discussed here builds on previous research on involving young people in systematic reviews (Jamal et al., 2014, Oliver et al., 2015).
How did we involve young people?
We began by identifying and contacting organisations who worked with young people affected by ACEs, as well as researchers and topic experts. We aimed to recruit between five and ten young people, knowing from previous experience that this was an optimum number to encourage everyone to talk. On the day, seven young people attended, most supported to do so by a mentor from the National Children’s Bureau.
At the workshop we spoke to the young people about our project. It was too early to relay concrete findings, but we told them about some emerging patterns in the research. As well as covering specific topics such as counselling and therapy (which dominates the effectiveness research), and discussing the kinds of outcomes they valued, the majority of the discussion took place around three main questions:
- What kinds of problems might children and young people affected by ACEs have?
- How might ACEs affect people differently (i.e depending on their gender, background, age)?
- What might help children and young people who are exposed to ACEs?
Our young advisors were very forthcoming in their responses to these broad questions as it provided a platform for everyone to contribute. In our original itinerary, we had allowed just ten minutes per question (out of a three-hour session) but we found that this activity was so fruitful and it provided such fertile ground for relevant discussions that we expanded the time allocation for this section. It was clear that the young people we consulted were already research literate and eager to contribute more. We thus discovered that allowing the group to explore these question was a better use of their time than talking through lots of detailed slides on concepts they were already familiar with.
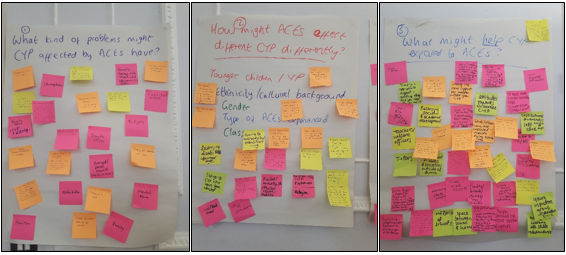
Key Learning Points
A thorough ethics application, co-creating ground rules, encouraging questions, and taking time to go through the consent form, were all essential to creating an atmosphere of mutual respect.
Being flexible and attentive to the individual preferences within the group maximised the usefulness of the workshop. Some young people were apprehensive about writing things down, preferring to talk out loud, whereas others found it easier to write their responses down on post it notes. We used a mixture of written and verbal, group and individual work in order to accommodate these various needs.
Being well prepared and knowing the material well helped us to move fluidly through the session.
We provided an information pack for the young people which we referred to throughout the session. It helped to give a sense of reassurance that the conversation could continue after the workshop and allowed more latitude for those working at different paces.
This echoes previous work by Dr Brady on involving young people with lived experience of substance misuse services: young people valued being able to use difficult personal experiences to create positive change, but doing so safely required being sensitive to individual circumstances and providing opportunities for young people who want to be involved to do so in ways that work for them (Brady et al., 2018).
Managing disclosure was also an important learning point. We explained to the young people early in the process that, although they were at the workshop because of their lived experience of ACEs, they did not need to share any personal details. However, several chose to do so, and this needed to be managed carefully to create a safe and comfortable space for everyone involved. Some of the young people who attended were also still going through difficult experiences and one needed time out because she was finding participation difficult. She left the session to sit in a nearby quiet room with the mentor who had accompanied her; and we made it clear she did not have to stay. But she chose to do so and re-joined the group for another section when she was ready. So some of our learning was around the need to expect the unexpected and be flexible in response to individual needs, particularly in this case when we were working with young people who had experienced, and in some cases were still living through, very difficult experiences.
How the workshop will inform the review
Two unifying themes of the discussion were the importance of the role of schools (even in regards to extended absence) and the need for support with practical life skills. The young people discussed how attitudes of teachers, institutional (in)flexibility, and (lack of) support at major transition points had been key barriers or facilitators to helping them feel supported through ACE trauma.
We are currently analysing primary qualitative UK studies in light of the themes arising from the workshop, with particular emphasis on the kinds of outcomes young people deemed to be important and the kinds of support that they highlighted which would help them to thrive.
The findings will be relayed to the Department of Health and Social Care and point to the need for them to work more closely with the Department of Education in their policy response to ACEs.
This is particularly relevant as the 2017 Green Paper sets out a vision to place schools at the foreground of mental health provision for children and young people (albeit by 2025).
Next Steps
As we near the final stages of the review, we are keen to re-engage with young people to verify the relevance of our findings across the different ACE populations and to the current UK context. There is also strong potential for further consultation with young people on the findings of the qualitative strand of the review, and for stakeholder events also including academics, practitioners and third sector organisations in order to reflect on or possibly to help disseminate findings.
About the authors
Dr. Louca-Mai Brady is an independent research consultant and a Research Associate at Kingston University and St George’s Joint Faculty of Health, Social Care and Education. Her research interests include children and young people’s involvement in health and social care research, policy and services and research with children and young people who are ‘less frequently heard’.
Sarah Lester is a Research Officer at EPPI-Centre. She is interested in conducting systematic reviews in the areas of mental health and social care and involving stakeholders in research processes.
This research is funded by the NIHR Policy Research Programme. Views expressed are those of the authors and not necessarily NIHR or the Department of Health and Social Care.
Bibliography
Allen, M., and Donkin, A. The impact of adverse experiences in the home on the health of children and young people, and inequalities in prevalence and effects. London: Institute of Health Equity: University College London, 2015.
Bellis, M., Hughes, K., Leckenby, N., et al. National household survey of adverse childhood experiences and their relationship with resilience to health-harming behaviors in England. BMC Medicine. 2014; 12, 72. https://bmcmedicine.biomedcentral.com/articles/10.1186/1741-7015-12-72
Brady, L., Templeton, L., Toner, P. et al. Involving young people in drug and alcohol research. Drugs and Alcohol Today 2018; 18 (1): 28-38. https://doi.org/10.1108/DAT-08-2017-0039
CDC. Centers for Disease Control and Prevention. Violence Prevention: ACE Study. https://www.cdc.gov/violenceprevention/acestudy/about.html (accessed 19.07.18)
Jamal, F., Langford, R., Daniels, P., et al. Consulting with young people to inform systematic reviews: an example from a review on the effects of schools on health. Health Expectations. 2014; 18 3225-3235. https://doi.org/10.1111/hex.12312
Kessler, R., McLaughlin K., Green J., et al. Childhood adversities and adult psychopathology in the WHO World Mental Health Surveys. The British Journal of Psychiatry. 2010; 197(5): 378-385. https://doi.org/10.1192/bjp.bp.110.080499
Oliver, K., Rees, R., Brady, L., et al. Broadening public participation in systematic reviews: a case example involving young people in two configurative reviews. Research Synthesis Methods. 2015; 6(2):206-217. https://doi.org/10.1002/jrsm.1145
Parsons, S., Thomson, W., Cresswell, K. et al. What do young people with rheumatic conditions in the UK think about research involvement? A qualitative study. Pediatric Rheumatology. 2018; 16 (35). https://doi.org/10.1186/s12969-018-0251-z
|
Graziosi posted on November 15, 2017 11:53
Dylan Kneale and Antonio Rojas-García reflect on recent work exploring the use of evidence in local public health decision-making. In new climates of public health decision-making, where the local salience of research evidence becomes an even more important determinant of its use, they question how much research is being wasted because it is not generalisable in local settings.
Our review on evidence use in local public health decision-making cast a spotlight on patterns and drivers of evidence use in England[1]. Locality was the recurring theme running throughout the review: evidence was prioritised where its salience to the local area was easily identified. Local salience included whether the evidence was transferable to the characteristics of the population, the epidemiological context, whether the proposed action was feasible (including economically feasible), but also included the political salience of the evidence.
To some, particularly those working in public health decision-making, these findings may feel all too familiar. The shift of decision-making to local government control in 2013 has served to increase the (local) politicisation of public health, which has consequent impacts on the way in which public health challenges are framed, the proposed actions to tackle these challenges, and the role of evidence in this new culture of decision-making. But these findings help to reinforce other lessons for generators evidence, because in many ways the review highlighted a thriving evidence use culture in local public health, but one that has a ‘make-do and mend’ feel about it.
Locality emerged as a key determinant of evidence use, but one where generators of evidence have only loosely engaged. There are likely good reasons for this, with research funders and publishers encouraging researchers to produce evidence that is relevant to audiences worldwide. However, this status quo means that in many ways, evidence generators may be guilty of adopting something akin to an ‘information deficit model’ around the use of research evidence – which inherently assumes that there is an information gap that can be plugged by ever more peer-reviewed research or systematic reviews that reflect ‘global’ findings[2]. This perspective overlooks epistemological issues around whether such knowledge is applicable, transferable, or otherwise relevant and useful in the context of local decision-making. It also assumes that decision-making is an event, rather than a cumulative learning process where outcomes are evaluated in different ways by different stakeholders; this perspective was reinforced in our own review by a paucity of studies that engaged with the nitty-gritty of public health decision-making processes.
The expression of a make-do and mend evidence culture is that public decision-makers working in Local Authorities take what they can from existing evidence sources (make-do), and plug the gaps with programs of local research and evaluation (mend), a finding that is also supported in later strands of our work (forthcoming). The fact that Local Authorities are undertaking their own research and evaluation activities to understand local people’s needs and preferences is, in many ways, to be commended. However, it also raises questions around the utility of public health research and how much public health research is being ‘wasted’[3], or rather its utility is not being maximised, through poor take-up in decision-making. Furthermore, our review shows that the methodological quality of this local research and evaluation activity is relatively unknown, and there exists a convention for much of this locally-conducted research to focus on public health needs and not on the effectiveness, or likely effectiveness, of action(s). Finally, an inward focus, with research being conducted in one site and not being shared across others, impedes on the dissemination of learning between other similar contexts.
So what’s to be done? As a principle, it is clear that there needs to be more regular dialogue between evidence generators/synthesisers and evidence users to understand decision-making needs and processes. New developments such as a dedicated journal on research stakeholder involvement are likely to advance our knowledge on effective ways of working and developing relationships across boundaries. But new methods are also needed to help understand the parameters of generalisability of research that is based on national or global level research and how (or if) it relates to local areas. We also need to strengthen our knowledge around what effective knowledge translation approaches look like. Our own review found that ‘experts’ were frequently deployed, potentially in a knowledge translation role, although their characteristics and precise function was unclear, and it is welcome to see a review further exploring knowledge translation competencies underway[4]. Finally we need to explore how we can better support Local Authorities to conduct the type of locally focussed research that they are currently producing, and appear to find the most useful, and to better understand the characteristics and features of this research. Supporting any one of these activities involves ensuring that funding exists, on both sides of the evidence generator/user divide, to foster relationships and to support the development of new methodological advances. But knowledge exchange should be integral to the role of all researchers working in public health. Social scientists in academia spend approximately 9 per cent of their time on knowledge exchange activities; in contrast we spend 23 per cent of our time on administration activities[5]! More of our time needs to be protected and spent on knowledge exchange activities if we are to better understand and respond to evidence needs in public health, and ensure that applied public health research can actually be applied.
The findings of our review reflected the literature on England, although devolution and localism in health and public health is a feature of many countries[6]. However, another trend is occurring in English public health that is likely to perpetuate a make-do and mend evidence use culture. Public health spending is shrinking with, for example, an expected £85 million slashed from sexual health and substance abuse by cash strapped Local Authorities in 2017/18 alone[7]. In times of shrinking budgets, the judicious use of evidence should become all the more important in offering greater access to information on what works, increased opportunities for the effective use of resources, and improved certainty around the likelihood of success. However unless we understand, and can better communicate, the generalisability of research evidence to decision-makers working locally, academic research evidence may fail to make the contribution to public health decision-making that it could, and indeed should, make.
About the authors
Dylan Kneale is a Research Officer at the EPPI-Centre. He is interested in developing methods to enhance the use of evidence in decision-making, focusing on demography, public health and social exclusion.
Antonio Rojas-García is a Research Associate-Systematic Reviewer at the UCL Department of Applied Health Research. Prior to joining UCL, he has been working on several research projects, mostly focused on health inequalities and health systems. Within UCL, he has been part of a number of projects exploring the use of research evidence, the impact of delayed discharges on patient, staff and health systems, among others.
Bibliography
1. Kneale D, Rojas-García A, Raine R, Thomas J: The use of evidence in English local public health decision-making. Implementation Science, 2017, 12(1):53.
2. Marteau TM, Sowden AJ, Armstrong D (eds.): Implementing research findings into practice: beyond the information deficit model. BMJ Publishing; 2002.
3. Wolfenden L, Ziersch A, Robinson P, Lowe J, Wiggers J.: Reducing research waste and improving research impact. Australian and New Zealand journal of public health, 2015, 39(4):303-4.
4. Mallidou AA, Atherton P, Chan L, Frisch N, Glegg S, Scarrow G: Protocol of a scoping review on knowledge translation competencies. Systematic reviews, 2017, 6(1):93.
5. Bullock A, Hughes R: Knowledge Exchange and the Social Sciences: A Report to ESRC from the Centre for Business Research. Cambridge: University of Cambridge; 2016.
6. Ham C, Timmins N: Managing health services through devolved governance: A perspective from Victoria, Australia. London: The King's Fund; 2015.
7. Gulland A. Spending on public health cut as councils look to save money. BMJ: British Medical Journal (Online), 2017 13:358.
Image Source (© 1939-1946, public domain).
|
Graziosi posted on November 09, 2017 11:00
Sandy Oliver discusses whether the worlds of academia and humanitarianism can combine to improve the delivery and understanding of the processes and benefits of humanitarian aid through use of evidence.
Interest is growing, when making decisions within the humanitarian sector, in drawing on systematic reviews of studies that assess the effects of different policies or practices. As in other sectors, such research evidence is considered alongside what else is known, such as about competing priorities, social norms, available resources or ease of implementing a programme. Professor Sandy Oliver argues that in contexts where rigorous studies of effects are few and far between, perhaps because conducting research is difficult in such circumstances, it is useful to learn from systematic reviews that encompass other forms of knowledge that are commonly held by individuals and organisations delivering humanitarian aid. These broader systematic reviews increasingly come from partnerships of academics and humanitarian organisations. Strengthening the links between research and work in the field helps create evidence-informed policy/practice, and policy/practice-informed evidence.
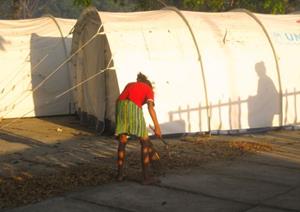
Finding evidence of effects
Humanitarian crises, whether ‘man-made’ or following natural disasters, seem overwhelming not only to the people caught up in them, but also to the individuals and organisations wanting to help. How do we know what will help? Humanitarian workers have first-hand experience. Well established organisations have tried and tested procedures. Researchers offer findings from studies designed to understand the nature and scale of the challenges being faced, to compare the effects of different programmes or systems, and to investigate how aid is delivered by organisations and individuals. This knowledge, whatever its source, is widely dispersed and can be difficult to find. One solution is to seek research findings to inform policy and practice by drawing on systematic reviews of research findings. There is growing enthusiasm for systematic reviews in the humanitarian sector. As with any new movement there are new challenges. We are encountering some of these challenges as we help to inform the efforts of humanitarian organisations and evidence champions who try to improve the lives of displaced populations in areas of conflict.
An immediate challenge for humanitarians is how to access systematic reviews quickly. We have found two particularly valuable types of resources: database of systematic reviews maintained by Evidence AID and 3ie; and evidence gap maps prepared by 3ie and the International Rescue Committee.
These resources signpost systematic reviews offering high quality evidence (often from controlled trials) of ways to improve access to health care, such as through involving communities in planning, implementing and or monitoring health service, or by training traditional birth attendants (Obuku et al., 2017). Similarly, we can find high quality evidence showing the benefits of cash or vouchers that give families access to food (Doocy and Tappis, 2016); and WASH (Water, Sanitation and Hygiene) interventions for reducing the spread of disease (Yates et al., 2017).
When evidence of effects is lacking
Particularly challenging, humanitarian organisations tell us, are the logistics and organisation required to deliver supplies and coordinate multiple agencies. Learning how to address these challenges requires different sorts of studies. Frustratingly, not only are there fewer systematic reviews addressing these challenges, but those that exist find relatively little relevant evidence.
In some cases systematic reviews tell us more about what not to do. Efforts addressing violence against women and girls have to be made in the absence of counterfactual studies offering evidence about the effects of prevention programmes. Instead, findings of observational or qualitative studies warn of potential harms. Livelihood programmes that raise women’s earned income may lead to increases in sexual violence, which is already common in areas of conflict. Women can experience: coercion by partners to hand over their earnings; increased risk when collecting firewood to sell; and insecure employment where lack of regulation exacerbates vulnerability (Spangaro et al., 2013). Where justice systems are weak encouraging survivors of sexual violence to testify can be counterproductive. Rather than perpetrators being convicted, survivors may experience retaliation, stigma and ostracism (Fulu et al., 2014). Although they do not cite the research, international guidelines recognise these challenges (Inter-Agency Standing Committee (2015).
In this way, where uncertainties about effective policies or practices are high, and the available research is meagre, learning has come from systematic reviews that address how organisations work, not only the effects of their work. For instance, where research has not addressed the effects of different models or mechanisms for coordinating humanitarian efforts (Akl et al., 2015), learning is available from a systematic review of studies describing how organisations have worked together (Lotfi et al., 2016). The most common model is clusters of national and international humanitarian agencies liaising around the main humanitarian sectors (such as food security, WASH or education) and within a country under the overall leadership of a humanitarian coordinator. They are designated by the Inter-Agency Standing Committee (IASC) and have clear responsibilities for coordination.
Knowledge from practice, organisational procedures and research
Conventionally research knowledge is seen as distinct from organisational or practice knowledge. However, the boundaries are becoming blurred as researchers involve humanitarian organisations in designing their studies, and conversely as humanitarian organisations take the lead in conducting or commissioning research. Examples of the former are the research team in Beirut systematically reviewing coordination of health services (Akl et al., 2015). They began their work by convening key stakeholders to discuss key questions needing answers. Similarly, reviewers addressing sexual violence in conflict and post-conflict zones convened an advisory group of consultants and programme directors with in-depth knowledge in the field. (Spangaro et al., 2013). Both reviews benefited from having direct involvement of people well-placed to inform the project and feed the findings directly into policy processes and practice. Examples of the latter are Oxfam and UNICEF. Both these organisations have commissioned or conducted systematic reviews to inform their own humanitarian work. Oxfam, in partnership with the Feinstein International Centre, has its own humanitarian evidence programme. UNICEF’s Baby Friendly Initiative is supported by systematic reviews of the effects of infant healthcare where much of the evidence comes from randomised controlled trials. In the area of peacebuilding, where RCTs are rare, UNICEF’s systematic review conducted in partnership with academics assesses how educational interventions may contribute to enhancing the agency of youth as peacebuilders (Lopes Cardozo et al., 2015).
Areas of work that are least developed have most to gain from systematic reviews conducted with or by humanitarian agencies who are able to draw on internal reports of the agencies themselves. This is well illustrated by UNICEF’s systematic review of humanitarian programming and monitoring in inaccessible conflict settings (Chaudhri et al., 2017). This review identified only 14 academic papers, largely observational studies. These were supplemented by many more organizational guidelines, programming notes, program evaluations, presentations, and meeting notes which, between them, offered concepts and definitions, and addressed the preliminary steps required to advance this burgeoning field.
Strengthening the evidence ecosystem
Evidence-informed humanitarian aid has had a low profile. Yet many of the essential components mentioned above are already in place. Systematic reviews are readily accessible through databases and evidence-gap maps. Guidelines are developed by consensus at international, regional and national levels. Academics and humanitarian agencies work together to pool resources from their different worlds. The next step to developing an evidence ecosystem for humanitarian aid may be strengthening the links between these components: guidelines informed by evidence; and humanitarian-academic partnerships shaping evidence syntheses. A likely mechanism for doing so could well be the existing system of clusters. The Inter-Agency Standing Committee already designates the clusters, and develops guidance for agencies working in the field and for inter-agency working. The potential for bringing evidence and guidance closer together is apparent in the area of gender based violence, where an overview of the evidence (The Global Women’s Institute and International Rescue Committee 2016) mentions the Inter-Agency Standing Committee (2015) guidance. An even closer relationship between guidance and evidence is illustrated by a systematic review in the area of mental health, (Bangpan et al., 2017) which employed the IASC’s (2007) intervention framework to present the available evidence. Lastly, the potential role for clusters is apparent from the systematic review of humanitarian work in inaccessible conflict settings, which was supported by the World Health Organisation as the Health Cluster Lead Agency and drew on guidance and research evidence (Chaudhri et al., 2017).
Conclusions
Learning how to deliver humanitarian aid, and about the effects it has, benefits from applying the principles of systematic reviewing to a broad range of documents that report research, policies, practices or discussions. Although the field of Evidence-based Humanitarian interventions is relatively young, all the basic components that can allow the emergence of a specialised evidence ecosystem are already in place. At this stage, it seems crucial to focus on inter-sector collaboration and cross fertilisation of ideas. In this way academics and humanitarian agencies working together can strengthen the links between their two worlds to develop further evidence-informed humanitarian aid.
About the image: Internally displaced person’s camp in Dili, Timor-Leste, 2006. © Anthony Zwi.
About the author
Professor Sandy Oliver is the Director of the Social Science Research Unit at UCL, and Deputy Director of the EPPI-Centre.
References
Akl EA, El-Jardali F, Bou Karroum L, El-Eid J, Brax H, Akik C, et al. (2015) Effectiveness of Mechanisms and Models of Coordination between Organizations, Agencies and Bodies Providing or Financing Health Services in Humanitarian Crises: A Systematic Review. PLoS ONE10(9). DOI: 10.1371/journal.pone.0137159.
Bangpan M, Dickson K, Felix L, Chiumento A. (2017). The impact of mental health and psychosocial support interventions on people affected by humanitarian emergencies: A systematic review. Humanitarian Evidence Programme. Oxford: Oxfam GB.
Chaudhri S, Cordes K, Miller N (2017) Humanitarian programming and monitoring in inaccessible conflict settings: A Literature Review. WHO - Health Cluster.
Doocy S, Tappis H. (2016) Cash-based approaches in humanitarian emergencies: a systematic review. 3ie Systematic Review Report 28. London: International Initiative for Impact Evaluation (3ie)
Fulu E, Kerr-Wilson A, Lang J. (2014) What works to prevent violence against women and girls? Evidence Review of interventions to prevent violence against women and girls. DFID, London.
Inter-Agency Standing Committee (2007) IASC Guidelines on Mental Health and Psychosocial Support in Emergency Settings. Geneva: IASC.
Inter-Agency Standing Committee (2015) Guidelines for Integrating Gender-Based Violence Interventions in Humanitarian Action: Reducing risk, promoting resilience and aiding recovery. Geneva: IASC.
Lopes Cardozo MTA, Higgins S, Maber E, Brandt CO, Kusmallah N, Le Mat MLJ. (2015) Literature Review: Youth Agency, Peacebuilding and Education, Research Consortium Education and Peacebuilding, University of Amsterdam.
Lotfi T, Bou-Karroum L, Darzi A, Hajjar R, El Rahyel A, El Eid J, Itani M, Brax H, Akik C, Osman M, Hassan G, El-Jardali F, Akl E. (2016) Coordinating the Provision of Health Services in Humanitarian Crises: a Systematic Review of Suggested Models. PLOS Currents Disasters. Edition 1. DOI: 10.1371/currents.dis.95e78d5a93bbf99fca68be64826575fa.
Obuku EA, Stewart R, Mijumbi R, Ochan M, Achana F, Akena D, Nakitende A, Ssemata A, Kinengyere A, Semakula D, Ssenono R, Nsangi A, Lalitha R, Mwesiga E, Akite J, Basaza R, Newbrander W, Okello J, Sewankambo N, with Dickson K, Oliver S. (2017) Working with non-state providers in post-conflict and fragile states in primary healthcare service delivery: a systematic review. London: EPPI-Centre, Social Science Research Unit, UCL Institute of Education, University College London.
Spangaro J, Zwi A, Adogu C, Ranmuthugala G, Davies GP, Steinacker L (2013) What is the evidence of the impact of initiatives to reduce risk and incidence of sexual violence in conflict and post-conflict zones and other humanitarian crises in lower and middle-income countries? A systematic review. London: EPPI-Centre, Social Science Research Unit, Institute of Education, University of London.
The Global Women’s Institute and International Rescue Committee. (2016) Evidence brief: What works to prevent and respond to violence against women and girls in conflict and humanitarian settings? Washington DC: George Washington University and London: IRC.
Yates, T., Allen, J., Leandre Joseph, M. and Lantagne, D. (2017). WASH interventions in disease outbreak response. Humanitarian Evidence Programme. Oxford: Oxfam GB.
|
Graziosi posted on November 08, 2017 14:18
Many humanitarians are evidence-aware, but may find it difficult to draw on what is known or find knowledge that speaks to their context. They may also be pressed for time to find or judge the relevance of what is often a dispersed literature. To address this gap the Humanitarian Evidence Programme, a partnership between Oxfam and Feinstein International Center at Tufts University, published eight systematic reviews in areas identified as a priority by humanitarian policy and practitioner stakeholders. Typical of the sector, and similar to international development, decision-makers ask very broad questions. Kelly Dickson and Mukdarut Bangpan reflect on the challenges we encountered when producing a mixed methods evidence synthesis for this programme, on mental health and psychosocial programmes for people affected by humanitarian emergencies.
Broad policy questions need comprehensive and flexible conceptual frameworks.
The humanitarian sector is a diverse field of inquiry. It can include earthquakes and typhoons, protracted conflict, acts of terrorism, biological hazards, and other natural and man-made disasters. Our brief, to consider the effectiveness and implementation of programmes addressing the mental health and psychosocial well-being of both children and adults, was similarly broad. To guide the review, we needed a comprehensive and flexible conceptual framework which was extensive enough to capture the diversity of definitions that can be found, but also specific enough to guide our reviewing task; from searching, screening, to shaping the synthesis. To achieve this, we drew heavily on existing research literature to provide us with widely recognised definitions of humanitarian emergencies and outcomes (e.g. post-traumatic stress syndrome, depression, anxiety, social support). We were also able to draw on existing guidelines on Mental Health and Psychosocial Support in Emergency Settings, which had benefitted from stakeholder input from United Nations agencies, NGOs and academic institutions. These guidelines provided an intervention framework, in the form of a 'layered system of MHPSS support', which was used to map and signpost studies in the review and supported useful identification of gaps in the evidence-base.
Emerging research areas need tailored search strategies…
Like for most systematic reviews we aimed to conduct a comprehensive search of the literature to identify the best available evidence to answer the review questions. We found that, similar to previous reviews, relying on bibliographic databases is not sufficient. Although some areas of research, such as the effects of clinical treatment, are well indexed in databases, other areas, such as studies of delivery and receipt of mental health programmes, were more often found by hand-searching, a time consuming but productive activity.
…and inclusive but transparent approaches to judging rigour and relevance.
One of the defining characteristics of systematic reviews is not only the transparent and accountable methods used to identify relevant literature, but the judgements that are made about the quality, and thus trustworthiness of the evidence to inform policy and practitioner decision-making. These judgements can be based on standardised tools for judging the methodological quality of evidence or those designed specifically for this review. A common criticism of systematic reviews, and a concern for the humanitarian evidence programme, was the potential to miss important lessons from studies of lower quality. Our approach was to two-fold. The first was to retain all studies, but to ‘grade’ the evidence informing the question on impact taking into consideration study quality rating, number of studies, and effect sizes, before making summary statements on the direction of effect (e.g. there is strong, moderate or limited evidence). The second was to judge the relevance of studies with qualitative evidence informing our question on implementation, clearly signposting this through the evidence synthesis. This approach, draws on our institutional learning, and addressed concerns from policy and practitioners keen to use evidence.
Systematic reviews shaped by humanitarian organisations are best shared through humanitarian organisations.
Not only did we draw on questions and concepts defined by humanitarian organisations, but we have also found them essential for sharing the findings. Our interpersonal relationships and networks (see Langer et al., 2016) have increased the visibility of the review findings. Through working closely with others as part of a ‘community of practice’ we were able to discuss the current evidence base and implications for the future, and found websites, conferences and webinars to suit wider policy and practice networks.
Conclusion
Producing mixed-methods evidence synthesis in a new arena such as the humanitarian evidence sector has presented us with an exciting opportunity to apply our skills and adapt synthesis methodology to benefit the field. This has required immersing ourselves in the policy literature to develop an appropriate conceptual framework, drawing on our technical expertise to identify and appraise studies and building new social networks to support dissemination activities. Doing so has, once again, enabled us draw on our insider knowledge of working at the interface of policy and practice to relevant and accessible evidence. The result is evidence where both relevance and rigour are maximised to suit decisions made in challenging circumstances.
About the authors
Mukdarut Bangpan is a research officer at the EPPI-Centre with many years' experience of conducting systematic review and supporting review teams, particularly in the area of international development.
Kelly Dickson is Research at the EPPI-Centre, experienced in conducting mixed methods systematic reviews and building research synthesis capacity across disciplines, including international development. She is also a psychotherapist with an interest in mental health and psychosocial wellbeing of adults and children.
Bibliography
Bangpan M, Lambert F, Chiumento A, Dickson K (2016). The impact of mental health and psychosocial support programmes for populations affected by humanitarian emergencies: a systematic review protocol. Oxford: Oxfam.
Interagency standing committee (2007). IASC guidelines on mental health and psychosocial support in emergency settings. Inter-Agency Standing Committee. https://interagencystandingcommittee.org/
Langer L, Tripney J, Gough D (2016). The science of using science: researching the use of Research evidence in decision-making. London: EPPI-Centre, Social Science Research Unit, UCL Institute of Education, University College London
|
Graziosi posted on February 10, 2017 17:15
The replication crisis, publication bias, p-hacking, harking, bad incentives, undesirable pressures and probably other factors all contribute to diminish the trustworthiness of published research, with obvious implications for research synthesis. Sergio Graziosi asks whether demanding simple theoretical clarity might be part of the solution.
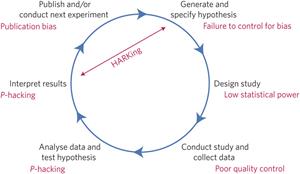
Within all the talk about publication bias, p-hacking, the replication crisis and so forth, I am finding it harder and harder to keep track of all the proposed solutions. While trying to organise my thoughts, I have realised that the absence of theoretical clarity underlies many of the problems that are currently being discussed. Perhaps this realisation is enough to justify a slight change in focus. For systematic reviewing, as Mark has reminded us, figuring out what results should be trusted, and perhaps more importantly, finding auditable and reasonably objective ways to do so is, naturally, of paramount importance. I do not think I need to convince anyone about this latter point, and will take it for granted in what follows.
More than ten years after warning us that most of the published research is false, Ioannidis (with colleagues) has produced a manifesto for reproducible science (Munafò et al. 2017). It is well worth a read, but it did not soothe my disquiet and confusion. On one hand, the manifesto comes with a range of concrete, actionable and agreeable suggestions. On the other, the same suggestions are, to my eyes, already worrying: the value of each remedial measure is likely to depend on how robust its implementation can be. Let’s consider pre-registration: it is a very attractive strategy and I am sure it is already contributing to diminish practices such as harking and p-hacking. However, on closer examination, one can find the contribution from Wicherts et al., where they list all degrees of freedom that researchers may exploit (consciously or not, legitimately or not) in their search for “significant” results. The list includes 34 potential problems, framing their discussion around the pitfalls that should be avoided when relying on preregistration. Ouch.
Checking for signs of 34 separate questionable practices when reviewing a single study in conjunction with its preregistration looks already daunting and close to utopian – especially when one remembers that the authors’ interest is to paint their own research in the most positive light. How many researchers are likely to critically consider each relevant pitfall of each step of their own workflow, and do so at the right time?
On the other side of the fence, to compile systematic reviews, one would need to go through the same checklist for all studies considered, and perhaps check the consistency of decisions across multiple reviewers. If I extrapolate, and assume that each of the twenty-plus strategies proposed in Munafò’s manifesto comes with a similar number of ways to fail to fully deliver its own potential (even if this doesn’t entail a combinatorial explosion, as there are many overlaps), my mind vacillates and immediately starts looking for strategies that come with lower cognitive costs.
What I will propose is indeed a shortcut. A (hopefully handy) heuristic that revolves around the role of theory in primary research. My starting point is a concise list of typical research phases (up-to and excluding research synthesis as such), being mindful that many alternatives exist. The table below may be read as a simplified version of the list produced by Wicherts et al., compiled with two underlying objectives: keeping it manageable, and highlight the role of theory. My main hunch is that when one clarifies the role played by theory in a given research phase, pitfalls, dangers and malpractice may become easier to isolate. You may decide to read what follows as an argument advocating for epistemological clarity in scientific reporting.
Research phase & role of theory |
Task |
Example(s) |
Pitfalls |
Counterarguments |
Theory building: this is typically done to try to accommodate the evidence that isn’t satisfactorily accounted-for by existing theories. |
Identify a need: as anomalies are accumulating, people start asking “do we need an entirely new theory?” |
Historically, theories such as electromagnetism. More recently, the creation of countless classifications of psychological ‘types’.
|
1. Fail to account for all/enough available evidence.
2. Fail to realise how current evidence may fit in existing frameworks.
3. Give new names/labels to existing concepts; fail to appreciate how existing theories use different labels to point at similar concepts or mechanisms.
4. Fail to capture regularities, which directly depend on non-contingent causal chains. |
1: No new theory can expect to account for all evidence from day zero.
2: That’s how theories degenerate: what if a new theory can accommodate more evidence with less ad-hoc extensions?
3: Existing theories are confusing, imprecise, too broad or too narrow.
4: This can be established only post-hoc. One needs to first theorise and then check that predictions do apply. Only then can one focus on causal explanations (secondary hypotheses).
|
Draft a new theory. |
Formulate new hypotheses: within a theoretical framework, many separate hypotheses can be identified. |
Data exploration: find patterns in existing data. |
Analysis and re-analyses of longitudinal studies |
1. Spurious correlations.
2. Pattern-finding bias (we tend to see patterns in noise).
3. Mistaking homogeneity for random noise (the opposite of pattern-finding).
4. Survivorship bias.
|
These pitfalls are irrelevant, because hypotheses need to be tested anyway. |
Theory-driven hypothesising. |
Deductively explore the consequences of a given theory, i.e. recalculation of expected light-bending effect of gravity as a result of general relativity. |
1. Logic failures and/or lack of imagination.
2. Overconfidence, producing hypotheses that are too far removed from tested-theory.
3. Lack of ambition: producing ever more detailed hypotheses, just to get publishable positive results.
|
1-2: as above.
3: this is how “normal science” is done!
|
Using theory |
Test an hypothesis. |
Measure the effect of a drug. |
1. Bad/insufficient clarity on what is tested.
2. Bad experimental design.
3. Low power.
4. Measure proliferation (encourages p-hacking).
5. Unpublished negative results / publication bias.
|
1: that’s science, people can’t know it all already.
2-4: budget/capacity. Science happens in the real world, we can do what we can do.
5: ditto, can’t spend ages trying to publish results that no-one wants to read.
|
Make predictions – applied science. |
Build bridges, design new microprocessors |
1. Overconfidence: stretching a theory beyond its known/expected limits of applicability.
2. Failure to account for theoretical boundaries (not knowing when/why a theory stops to apply).
3. Failure to measure outcomes.
|
1: But, but, science told us it will work!
2: Can’t anticipate unknown unknowns.
3: We don’t need to, because our theory is solid.
|
The interesting part of this exercise is how many of the known problems are not, or are only marginally captured by the table above – I would argue that a good number fall in the cracks between the cells above. Thus, my point is that clarifying what one is doing (am I producing a new hypothesis? Am I testing a given one? Am I trying to see if we should start looking for new possible theories?) should be second-nature for all scientists (but alas, I don’t think it is). This may make it easier to double check for well-known pitfalls, but also to avoid stumbling on the boundaries between separate tasks. For example, P-hacking and HARKing can be deliberate malpractice, or could result from “Bad/insufficient clarity on what is tested”. However, it seems to me that it may also be caused by a lack of separation between hypothesis testing and data-exploration.
For example, we may imagine our typical scientist: in this imaginary scenario, her normal work starts by testing a hypothesis – to keep it simple, we’ll assume she is testing the effectiveness of drug D. Let’s imagine she finds a tiny effect size, but with a considerable number of outliers which seem to be clustered together. The original experiment was testing a hypothesis: first result is that drug D doesn’t appear to work. However, we now have new data to explore (a different task), and perhaps we can find that the outliers all have trait T in common. The same experiment therefore yielded a second (separate!) result: we now have a new hypothesis - perhaps drug D only works on subjects with T.
One study yielded two “results”, one is negative or inconclusive; the second is a new hypothesis. Being a new hypothesis, it needs to be tested. In our oversimplified example, the data suggests a new hypothesis, and therefore it can’t also confirm it.
In other words, perhaps we can agree that clarifying and segregating tasks based on how they relate to theory has helped identifying a well-known problem with unreliable science, and has concurrently made it clear how to best use the data collected. Most scientific work actually happens across multiple phases, but nevertheless, having a clear conceptual picture of the boundaries could be a useful approach to avoid repeating well-known mistakes. It goes without saying that such conceptual clarity, if transferred in published research articles, also has the potential of making the task of systematic reviewers less prone to error and less dependent on hard to audit personal judgements.
Is this simplistic proposal enough to overcome all the problems mentioned above? Of course not. It is a mere heuristic; a simple rule of thumb, which I hope might be useful to our readers. If time permits, I hope to explore this same theme in the context of conducting systematic reviews in a follow-up post. In my studies (molecular biology and neuroscience), no one ever helped me realise how the role of theory relates with the different mistakes that may plague scientific results. In fact, no one ever discussed the epistemological foundations of science; I guess they were mostly taken for granted. Thus, perhaps my suggestion is directed to educators in particular: discussing and clarifying the distinctions I’ve mentioned here might be a low-cost strategy to help the next generation of scientists not to repeat our own mistakes.
About the author
Sergio Graziosi is the EPPI-Centre IT manager, and one of the developers of EPPI-Reviewer. Designing tools to conduct systematic reviews implicitly requires exploring what can and cannot count as good/reliable evidence. As a consequence, he’s been exploring science epistemology on his own (non-academic) blog.
Bibliography

Kerr NL (1998). HARKing: hypothesizing after the results are known. Personality and social psychology review : an official journal of the Society for Personality and Social Psychology, Inc, 2 (3), 196-217 PMID: 15647155
Head ML, Holman L, Lanfear R, Kahn AT, & Jennions MD (2015). The extent and consequences of p-hacking in science. PLoS biology, 13 (3) PMID: 25768323
Ioannidis JP (2005). Why most published research findings are false. PLoS medicine, 2 (8) PMID: 16060722
Munafò, M., Nosek, B., Bishop, D., Button, K., Chambers, C., Percie du Sert, N., Simonsohn, U., Wagenmakers, E., Ware, J., & Ioannidis, J. (2017). A manifesto for reproducible science Nature Human Behaviour, 1 (1) DOI: 10.1038/s41562-016-0021
Wicherts JM, Veldkamp CL, Augusteijn HE, Bakker M, van Aert RC, & van Assen MA (2016). Degrees of Freedom in Planning, Running, Analyzing, and Reporting Psychological Studies: A Checklist to Avoid p-Hacking. Frontiers in psychology, 7 PMID: 27933012
Image Credits: © Munafò et al., Nature Publishing Group (CC-BY).
|
|
|
|
 |
Note: Articles on the EPPI Centre Blog reflect the views of the author and not necessarily those of the EPPI Centre or UCL. The editorial and peer review process used to select blog articles is intended to identify topics of interest. See also the comments policy.
|
|
|
|
|
|
|
|
|