One of the most powerful new features available is the ability to keep a review up to date using the OpenAlex dataset.
Every two weeks a new copy of the database arrives, with up to around a million new records. If your review is ‘subscribed’ to the auto-updating service, a machine learning model will ‘learn’ the scope of your review, and automatically suggest new records that might be relevant. You subscribe to this service on the ‘keep review up to date’ tab, selecting whether the machine learning model should analyse ALL the items in your review, or only those with a specific code. Most reviews will need to select only items with a specific code, as your review probably contains both items that are, and are not, relevant to your review. It is important to ensure that the machine ‘learns’ only from those items that are actually relevant to your review.
Each time the service runs, a new row will appear in the list entitled ‘Items found at each task execution’ with the most recent listed at the top. You can then click on the ‘refine / import’ link to decide what to do with the new items that the machine learning service has identified.
As the Figure below shows, you use the options available on the ‘refine / import’ page to determine which items you want to bring into your review. The first graph that appears here are the results from the ‘auto-update’ algorithm. This is the algorithm that examines all the newly arrived records and determines whether any of them might be relevant for your review. It is a very sensitive algorithm, and so will probably produce many more candidate records than you want to examine; its purpose is to find all potentially relevant records and leave fine-tuning to subsequent steps.
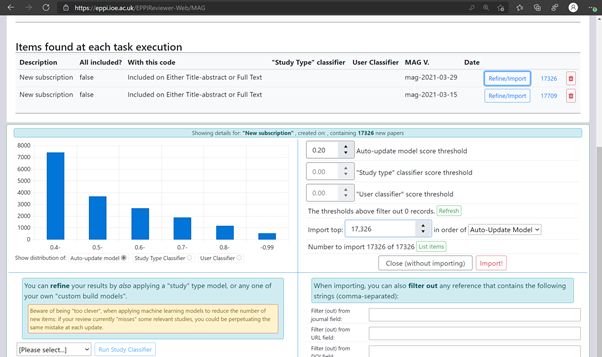
These subsequent steps involve one or more of the following:
- importing the top n records according to the above algorithm (where ‘n’ is a number determined by each review independently depending on screening capacity and previous evaluation of the use of the auto update scores
- using one of EPPI-Reviewer’s study type classifiers either to rank records according to their relevance, or to use a cut-off threshold, below which records will not be imported
- using a ‘user’ classifier, which is a classifier built using review data
For example, a workflow that aims to identify randomized controlled trials that evaluate smoking cessation interventions might classify all records using the RCT classifier, and also all records using a user classifier that was built using the included and excluded studies from the original review. It would set a cut-off on the RCT classifier and exclude all records that fall below a given threshold (e.g. 0.1) and then import the top n items ranked according to the user classifier. This would maximise the chances of identifying relevant records by filtering out all records that were clearly the wrong study type, and then importing those that were most likely to be relevant to the review according to a classifier built using its data. The review authors might then use priority screening to screen the resulting records (though would aim to keep them to a reasonably small number, as they could be importing similar numbers of records every two weeks).
If you are not looking for RCTs (or systematic reviews or economic evaluations), you have two choices. You can either import the very highest scoring items in the list into your review, or you can run a ‘user classifier’ that can be built using your existing include / exclude decisions. We recommend that you experiment with ranking new records by auto update and user classifier scores and examining which thresholds might work best for your particular use case. It’s likely that a combination of setting a threshold (e.g. 0.5) on the auto update model score and then ranking the records according to your user classifier will give you the best results.
Please bear in mind that this is a very new feature, and methods and tools are still developing. We are therefore being far too over-inclusive in the number of records retrieved at the moment. In the example shown in Figure 2, there are about 17,000 new candidate records arriving in that review. Bearing in mind that these kinds of numbers arrive every two weeks, we are not suggesting that they all need to be looked at. The aim of the over-inclusivity is to enable experimentation using the study type and user classifiers, so please don’t be put off by the dauntingly large numbers of records being identified! Our aim is to support methods development here, and it is unlikely that more than a handful of relevant records are published each week: the handful of records is likely to be in the set retrieved, and the ranking algorithms aim to bring them to the top of the list.